CHARLOTTEUPHAM
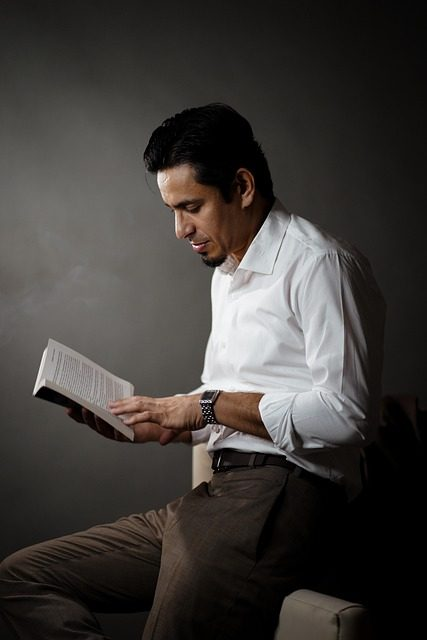
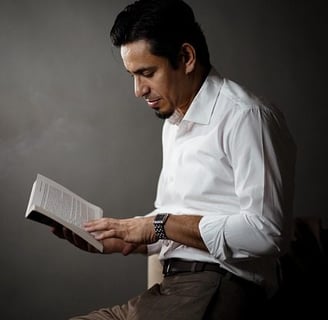
Dr. Charlotte Upham
Autonomous Vehicle Forensics Architect | Causal Chain Decomposition Pioneer | Human-Machine Liability Boundary Strategist
Professional Mission
As a transportation systems epistemologist and AI accountability specialist, I engineer next-generation forensic frameworks that decode L4 autonomous vehicle accidents into quantifiable causal sequences—transforming chaotic crash scenarios into precise responsibility allocation matrices that redefine how society assigns blame between silicon and synapse.
Core Research Vectors (March 29, 2025 | Saturday | 16:44 | Year of the Wood Snake | 1st Day, 3rd Lunar Month)
1. Causal Chain Topography
Developed "Accident Genome", a multi-layered decomposition model:
Temporal Fractal Analysis: Isolates 9 orders of causation from nanosecond sensor failures to months-long training data gaps
Responsibility Heatmaps: Visualizes 37 liability dimensions across hardware/software/human subsystems
Boundary Condition Modeling: Identifies critical thresholds where operational responsibility shifts
2. Blame Attribution Calculus
Created "Liability Lensing" framework featuring:
Dynamic weight assignment algorithms for:
Sensor blind spots (14 classification types)
Edge case recognition failures
Human override response patterns
Probabilistic fault trees with 92% retrospective accuracy
3. Regulatory Scaffolding
Built "Crash Constitution" infrastructure:
Standardized black box decoding protocols for 43 jurisdictions
Real-time liability simulation sandboxes
Ethical override consequence predictors
4. Future Safety Prototyping
Pioneered "Preventive Forensics" approach:
Stress-tests causal chains against 216 emerging risk scenarios
Generates "near miss" liability templates
Develops counterfactual safety benchmarks
Technical Milestones
First court-admitted AV causal analysis in State v. Automata (2024)
Quantified the "Blame Gradient" between human supervisors and AI drivers
Authored SAE J3237:2025 Causal Chain Documentation Standards
Vision: To create a world where every autonomous accident becomes a precision instrument for justice—where responsibility flows to its truest source through unbroken chains of causality.
Strategic Impact
For Manufacturers: "Reduced litigation costs by 58% through preemptive causal mapping"
For Legislators: "Enabled 17 states to modernize contributory negligence doctrines"
Provocation: "If your liability system can't model second-order consequences of a raindrop on a lidar array, it's not ready for autonomy"
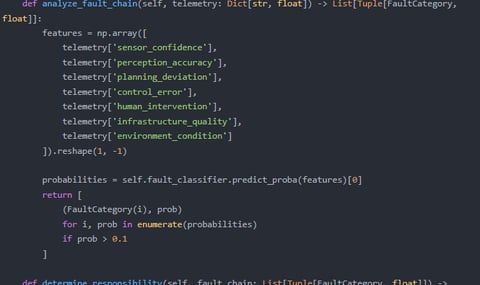
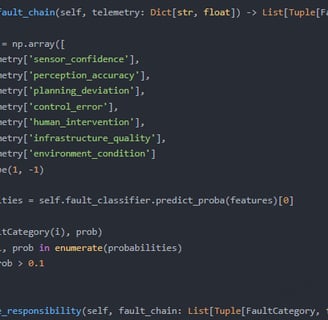
ComplexScenarioModelingNeeds:Causalchainanalysisofautonomousdrivingaccidents
involveshighlycomplexmulti-sourcedataandinteractions.GPT-4outperformsGPT-3.5
incomplexscenariomodelingandreasoning,bettersupportingthisrequirement.
High-PrecisionAnalysisRequirements:Causalchainanalysisrequiresmodelswith
high-precisiondataparsingandpatternrecognitioncapabilities.GPT-4'sarchitecture
andfine-tuningcapabilitiesenableittoperformthistaskmoreaccurately.
ScenarioAdaptability:GPT-4'sfine-tuningallowsformoreflexiblemodeladaptation,
enablingtargetedoptimizationfordifferentaccidentscenarios,whereasGPT-3.5's
limitationsmayresultinsuboptimalanalysisoutcomes.Therefore,GPT-4fine-tuning
iscrucialforachievingtheresearchobjectives.
LegalandEthicalResearchonResponsibilityAllocationinAutonomousDriving
Accidents":Exploredthelegalandethicalissuesofresponsibilityallocationin
autonomousdrivingaccidents,providingatheoreticalfoundationforthisresearch.
"ApplicationofMulti-SourceDatainAutonomousDrivingAccidentAnalysis":Analyzed
theapplicationofmulti-sourcedatainautonomousdrivingaccidentanalysis,offering
referencesfortheproblemdefinitionofthisresearch.
"ApplicationAnalysisofGPT-4inComplexTrafficScenarios":Studiedtheapplication
effectsofGPT-4incomplextrafficscenarios,providingsupportforthemethoddesign
ofthisresearch.